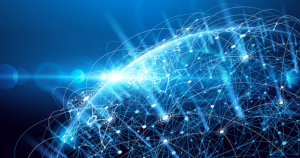
It was inevitable that artificial intelligence technology would eventually be implemented into cyber defense (and hacking, for that matter). What was less obvious was how exactly that implementation would occur. Now that the shift has begun, we’re developing a greater understanding.
AI is particularly adept at handling the large volumes of data that flow through an IT network. A corporate IT infrastructure–or, frankly, even a relatively small business network–will see a lot of information pass into and out of the borders of the network. That data is, overwhelmingly, legitimate traffic. Though sometimes it is suspicious. How do we spot unauthorized access? Well, we have firewalls and packet sniffers and, if all else fails, a person can manually inspect the traffic themselves.
These methods, while useful, have clear and obvious flaws. The manual labor necessary in actively inspecting network traffic is both costly and tedious–akin to finding a needle in a haystack, when you don’t know if there’s a needle to be found in the first place. Furthermore, malicious actors routinely bypass standard IT defenses.
We can look to any number of past failures to see how this plays out. Consider the 2017 breach of Equifax. When the personal data of over one hundred million Americans was lost to an unknown entity, the public blamed the company’s executives for failing to take security seriously enough. In fact, Equifax had invested millions of dollars into its own dedicated cyber operations center, with a bevy of high-end security programs at its disposal.
One of those programs was Moloch, a tool for recording network traffic. After the dust settled, Moloch was how investigators pieced together the breach, and learned what went wrong along the way.
While the attackers’ initial entry point was, in fact, a blatant security oversight–unpatched software that allowed for the installation of a malicious web shell–the majority of the attack sequence had to do with bypassing firewalls, forgoing network security protocols and installing special-access tunnels from one area of the network to the next. The criminals were so advanced, so well-coordinated, that they were able to move into any corner of Equifax’s network that they wanted, bypassing every bit of the company’s fancy firewalls and anti-intrusion protections. At no point did Equifax employees properly understand what was going on right under their noses.
AI is particularly adept at the kind of pattern recognition necessary to sussing out malicious network traffic. In fact, at its core, AI is just pattern recognition software. It trains on existing data to heighten its senses as to what constitutes legitimate, and illegitimate, network activity.
We know that Equifax employed AI to analyze customer data, but we don’t know whether they deployed it as part of their security infrastructure. What we can say is that AI-driven network monitoring is designed to combat exactly the kind of malicious activity that Equifax experienced during those months.
Say, hypothetically, that the company had purchased advanced AI monitoring in the years leading up to their hack. The software would have been able to observe the network over many months, gradually picking up on potentially suspicious activity, and correcting for false red flags. By learning about the patterns of activity common over the network–which accounts accessed which data sets, when users were logging in and how they interacted with one another, and so on–the algorithm could have built a broad predictive model of what constitutes “normal” network behavior.
By the time any hackers arrived on an AI-protected Equifax network, their presence would have been glaringly obvious. Even after they hacked into legitimate accounts, the algorithm would be able to spot their unusual activity–that those accounts typically didn’t do what they were now being asked to do. A red flag would pop up at the cyber defense center, and the threat could be nipped before developing into a crisis situation.
Perhaps you’re thinking: if the Equifax hacker was able to bypass every advanced security measure the company had in place, who’s to say AI would have stopped them?
There is, of course, no way to rewrite history and prove anything. However, unlike firewalls and anti-intrusion software–of the kind Equifax’s hackers successfully bypassed–there is an intrinsic characteristic to AI that makes it very difficult to get around.
The “black box problem” is a phenomenon common across all advanced machine learning algorithms. Because AI learns to make its own decisions, and those decisions are informed by extremely deep, complicated and interconnected mathematical functions, it can be impossibly difficult to determine exactly how an algorithm reaches certain conclusions.
The black box problem can be a headache for security professionals, because it limits their control over their own tools. On the flip side, however, the black box problem is an even bigger barrier for hackers. Think about it: if there’s no way to predict exactly what spurs an algorithm to arrive at one conclusion or another, no hacker can reliably build a method of subverting that algorithm. The Equifax hackers knew exactly how to tunnel in and around Equifax’s network security measures, because those security measures were static, and well-understood. Each artificial intelligence algorithm is unique, constantly evolving, and impossible to know in full. And no attacker can reliably defeat what they cannot reliably understand.
This is the power of AI. In the coming years, this power will become more and more evident with each new threat actor that’s stopped in their tracks.
About the author:
Nathaniel Nelson writes the internationally top-ranked “Malicious Life” podcast on iTunes, hosts programs on blockchain and SCADA security, and contributes to AI and emerging tech blogs.